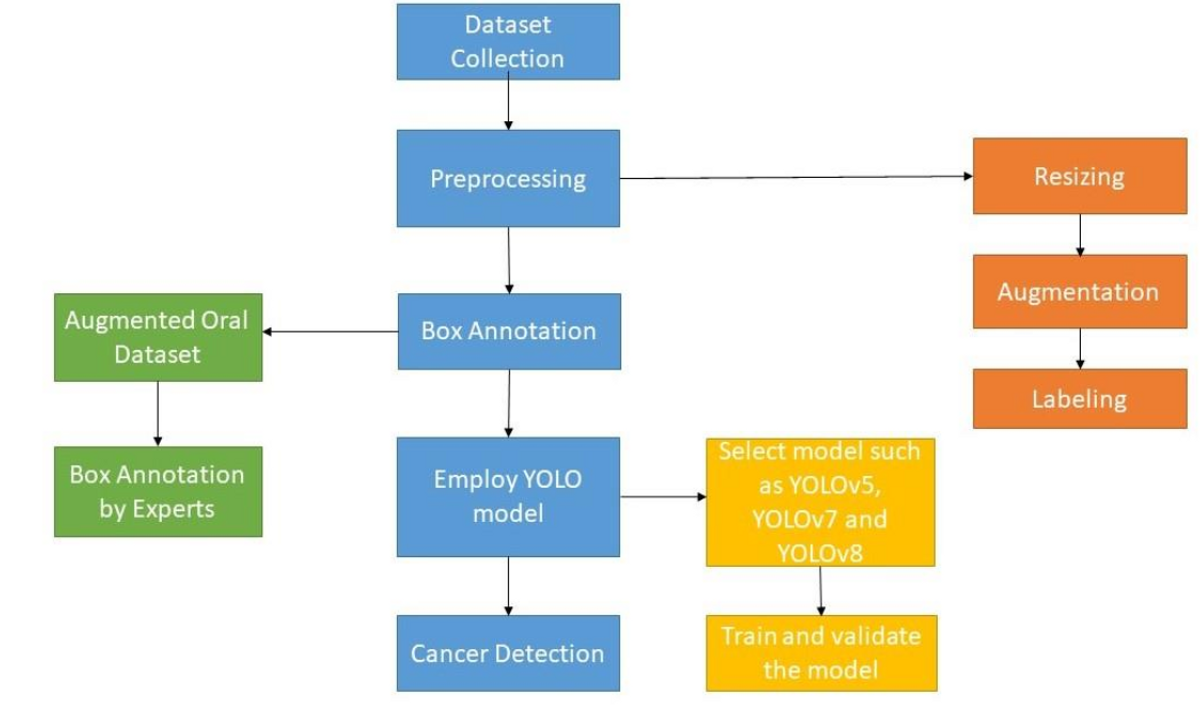
Oral Carcinoma Detection for Custom Dataset using Box Annotation with YOLO Model
ral carcinoma is a significant public health concern with a high mortality rate that affects millions of people worldwide. Early detection plays a pivotal role in ensuring the efficacy of treatment and improves survival rates. The field of deep learning has surfaced as a highly encouraging methodology for automated oral cancer detection. The primary goal of this research is to achieve heightened accuracy in cancer detection by integrating bounding box annotations into two distinct datasets: one comprising collected clinical data and another featuring benchmark data of oral carcinoma. The aim of this study is to advance the state of oral cancer detection using deep learning techniques, specifically YOLOv5, YOLOv7 and YOLOv8. The proposed methodology encompasses data collection, preprocessing, expert-driven bounding box annotation and the application of state-of-the-art deep learning models, YOLOv5, YOLOv7 and YOLOv8, for lesion detection. YOLOv5, YOLOv7 and YOLOv8, recognized for their efficacy in object detection tasks, are employed to precisely identify cancerous regions within oral images. Bounding boxes provided by experts in the field are strategically added to facilitate the nuanced pinpointing of these regions. Expert annotations in the form of bounding boxes are meticulously added to pinpoint cancerous regions within the images. The models are trained and fine-tuned on the annotated dataset, leveraging their ability to precisely locate and categorize cancerous regions. Subsequently, a comprehensive evaluation is conducted, measuring the performance of both models based on precision, recall rate (sensitivity) and F1 score. The YOLOv8 model outperforms other models. This research demonstrates the promising potential of AI-based object detection models to revolutionize oral cancer screening and diagnosis, paving the way for more efficient and accurate early detection, ultimately improving patient care and outcomes.
Research Images
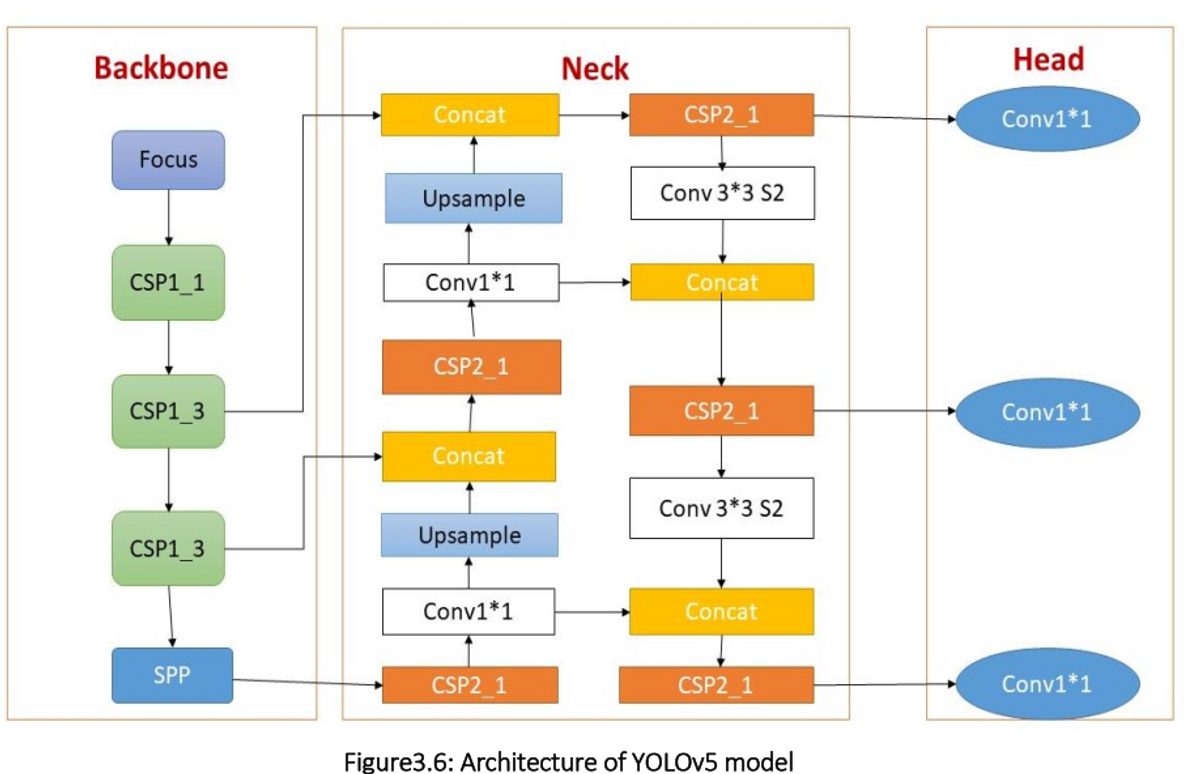
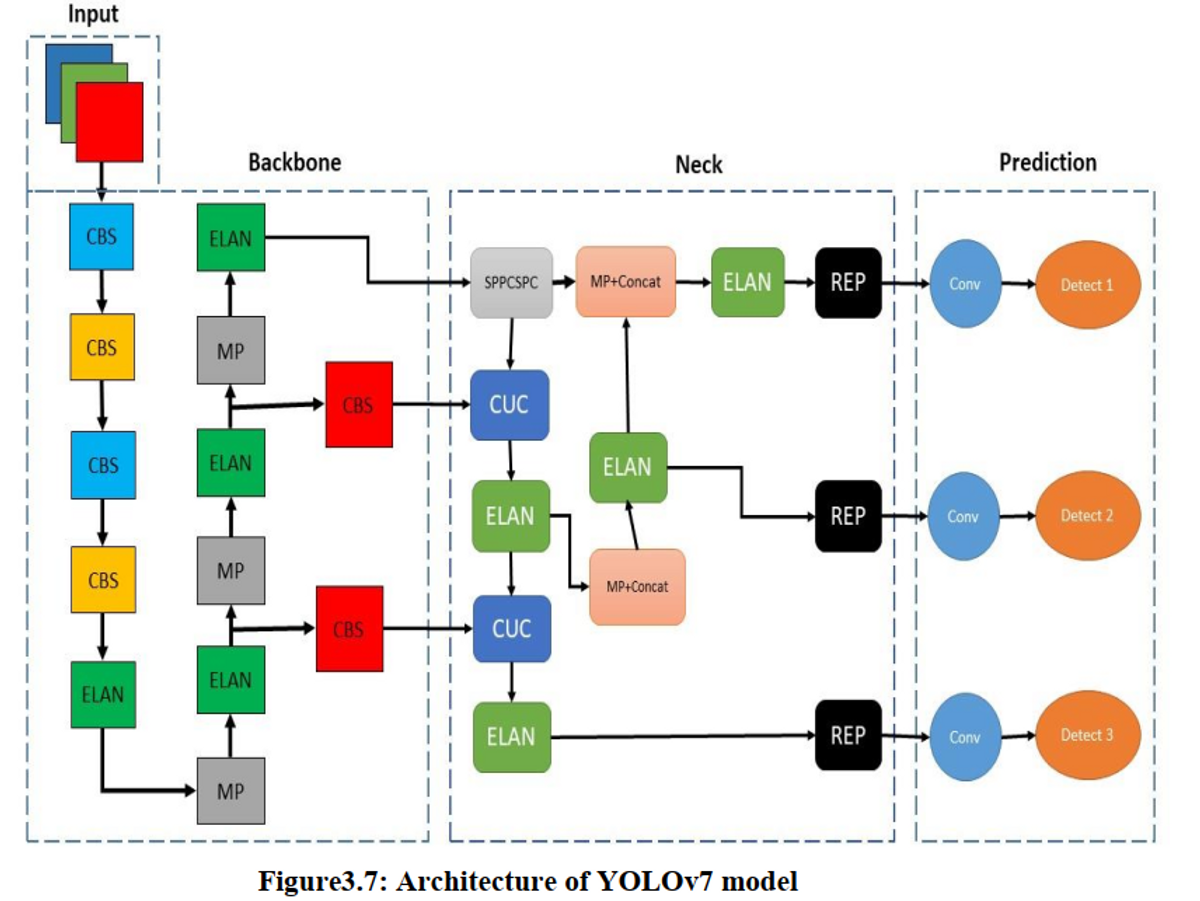